How Data Analytics Are Used in Marketing
A closer look into three main kinds of data-driven marketing analytics – descriptive, predictive and prescriptive.
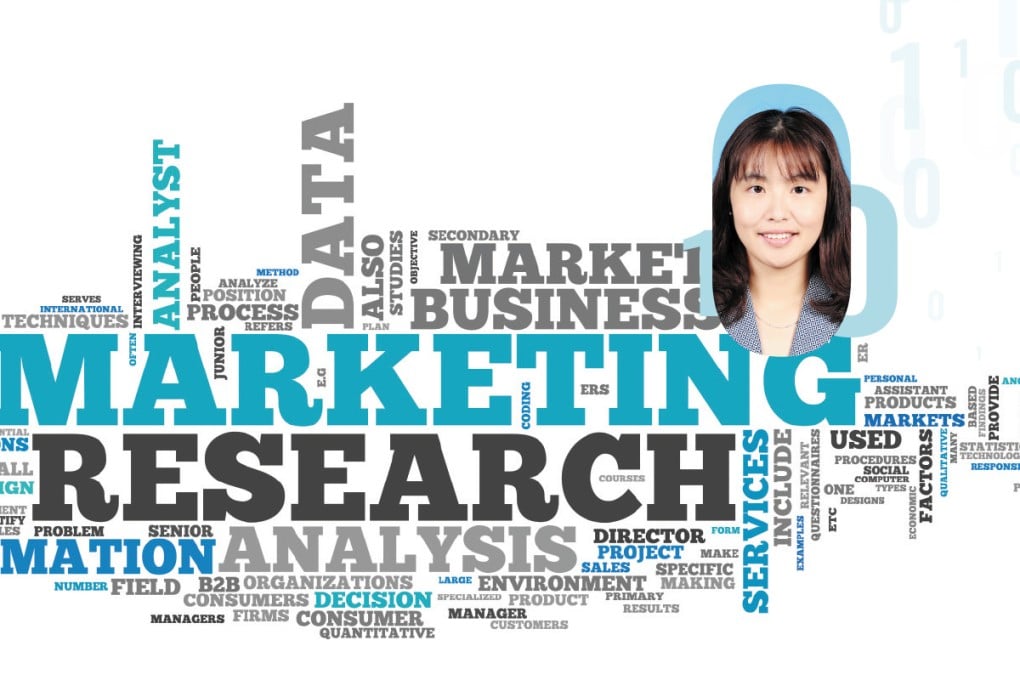
[Sponsored Article]
Data-driven marketing simply put means using quantitative methods to derive meaning from data to make informed marketing decisions. Thanks to the availability of a massive amount of data, data analytics in marketing has become more important than ever. According to a BMO Capital Markets report, marketers spend US$50 billion per year on big data and advanced analytics to improve marketing’s impact on business. Research by McKinsey1 shows companies that invest in big data and analytics yield a five to six percent average increase in profits, which jumps to nine percent for investments spanning five years.
In many cases, companies have focused on more open-ended efforts to gain novel insights from big data. These efforts were fuelled by analytics vendors and data scientists who were eager to take data and run all types of analyses in the hope of finding diamonds. Depending on the stage of the workflow and the requirement of data analysis, there are three main kinds of data driven marketing analytics – descriptive, predictive and prescriptive. Their underlying techniques include, but are not limited to, statistical modelling, data mining, machine learning, and AI.
In this article, I will present some applications of each of the three analytics using recent research in marketing, most of which reflect my own (working) papers on marketing analytics.
Descriptive Analytics
Descriptive analytics refers to the interpretation of historical data to identify trends and patterns, answering the question “What has happened?”. This means descriptive analytics can help identify potential problems or future opportunities for business.
In my collaboration with the Microsoft Bing search engine2, we have developed an interpretable machine learning model that can track, quantify, and interpret users’ topical preferences underlying each search query and across search contexts (e.g., time, location, and demographics). The proposed model leverages data on user queries, subsequent click-through on search results, and all textual information encountered on the search engine. The outputs of our proposed model can help search engines (advertisers) improve their search results (keyword and ad copy strategies).