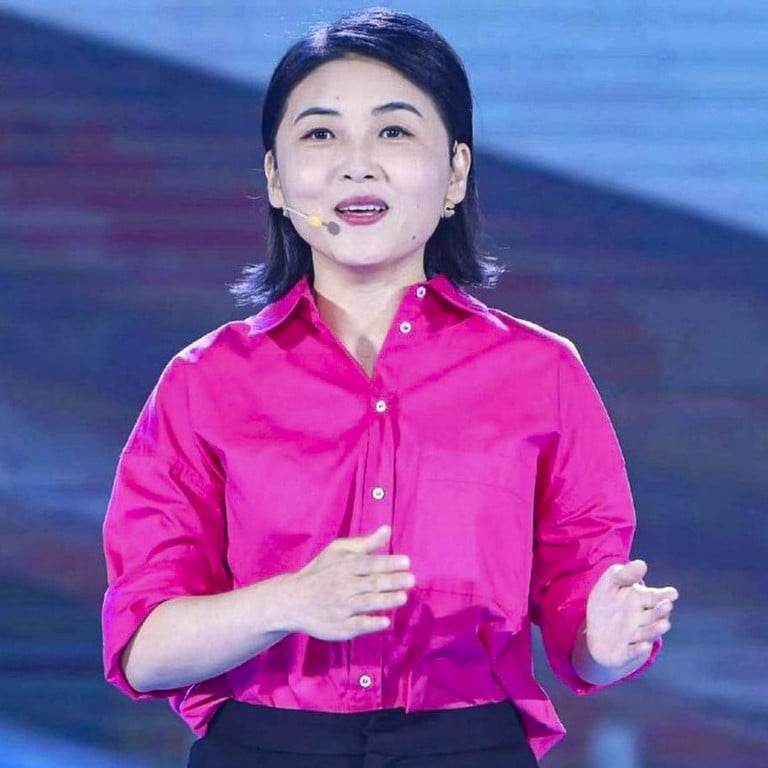
Chinese scientist Yan Ning denies AI took her job in US and forced her back home
- Structural biologist tells Shenzhen forum there remain many places AI has not reached in her field of research
- Expert says AlphaFold algorithm has uses but he remains concerned about its role in development of new drugs
Structural biologist Yan Ning said there were still many places artificial intelligence had not reached in her field of research. “We embrace AI, but we are disappointed after trying it out,” said Yan, who is also known as Nieng Yan.
Yan made the assessment at the Xplorer Forum hosted by the Southern University of Science and Technology in Shenzhen on November 27.
Yan, who left Tsinghua University for Princeton in 2017, declared on November 1 she had quit her job in the US and would establish and serve as dean of Shenzhen Medical Academy of Research and Translation.
Her Xplorer speech was in response to online claims that she chose to return to China because the development of AI systems such as AlphaFold had created her “unemployment”.
“We are excited when we find AlphaFold can predict 3D structures of proteins with their amino acid sequence. But we are looking beyond the basic structure, we resolve protein structures to find their applications in drug development,” she said.
“When I checked the sodium ion channels in the structure, it turns out AlphaFold only reached what my team achieved in 2017. This is no surprise … my team is the only group that is researching on the topic of sodium ion channels, and I think AlphaFold is making predictions based on the structure we published.
“Since we are providing the database for the training of AlphaFold, it is hard for AlphaFold to go beyond us and make new predictions. I realised this limitation for AlphaFold in August 2021. When I checked the database again yesterday, the predictions in sodium ion channels have not been updated yet.”
The speech reveals a main concern about the limits of AI in structural biology research. For some top scientists, the subject of structural biology is biology – to understand life and make biological discoveries – and resolving structures is merely a method used to answer questions.
A spokesperson for DeepMind said on Tuesday: “Our AlphaFold Protein Structure Database has already empowered over 750,000 researchers and biologists in over 190 countries to accelerate new areas of research in a whole range of fundamental and complex fields.
“AlphaFold was designed as a state-of-the-art protein structure prediction tool to accelerate the work of scientists. Experimental structure determination conducted by scientists remains extremely valuable.”
China-US divide extends to AI, both in research and real life: study
AI does speed up the progress of some traditional research. What AlphaFold can accomplish in minutes – if not seconds – could have taken years in the past for researchers using traditional X-ray crystallography. But the universality and accuracy of the algorithm are not always satisfying.
Computational biologist Professor Ma Jianpeng, of the Multiscale Research Institute for Complex Systems at Fudan University, said techniques like AlphaFold were powerful, but far from replacing experimental approaches.
“AlphaFold2’s algorithm significantly outperformed other teams at CASP14, the Olympiad of protein structural prediction in December 2020, but when predicting protein structures for the human proteome in 2021, only 35.7 per cent of its prediction fall within the highest accuracy band,” Ma said.
“The result is not bad, but not enough to replace scientists across the board.
“AI predictions are based on its learning from known protein structures, so it is more exact for proteins with similar amino acid sequences and seems flawed for unfamiliar proteins. Much work still needs to be done.”
“Scientists should be cautious when using [the] AlphaFold prediction, because we found that there is a gap between the predicted structure and the real experimental data. This gap could be magnified thousands of times in pharmaceuticals,” Yan told the Xplorer Forum.
US unveils artificial intelligence ‘Bill of Rights’ to safeguard civil rights
Ma said: “Predictions of protein side chain are important in the development of new drugs, since the binding of drug molecules to proteins is mostly achieved by interacting with side chain. But AlphaFold is not accurate enough for mechanistic study or ligand design in side-chain predictions.”
However, Yan is positive about the future of AI. “True researchers are willing to embrace technological progress, and should be good at using various technologies to explore and answer questions they are interested in. I expect AI to become stronger and stronger,” she said.
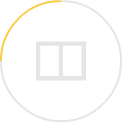